Imaginary worlds dreamed by BigGAN
These are some of the most amazing generated images I’ve ever seen. Introducing BigGAN, a neural network that generates high-resolution, sometimes photorealistic, imitations of photos it’s seen. None of the images below are real - they’re all generated by BigGAN.
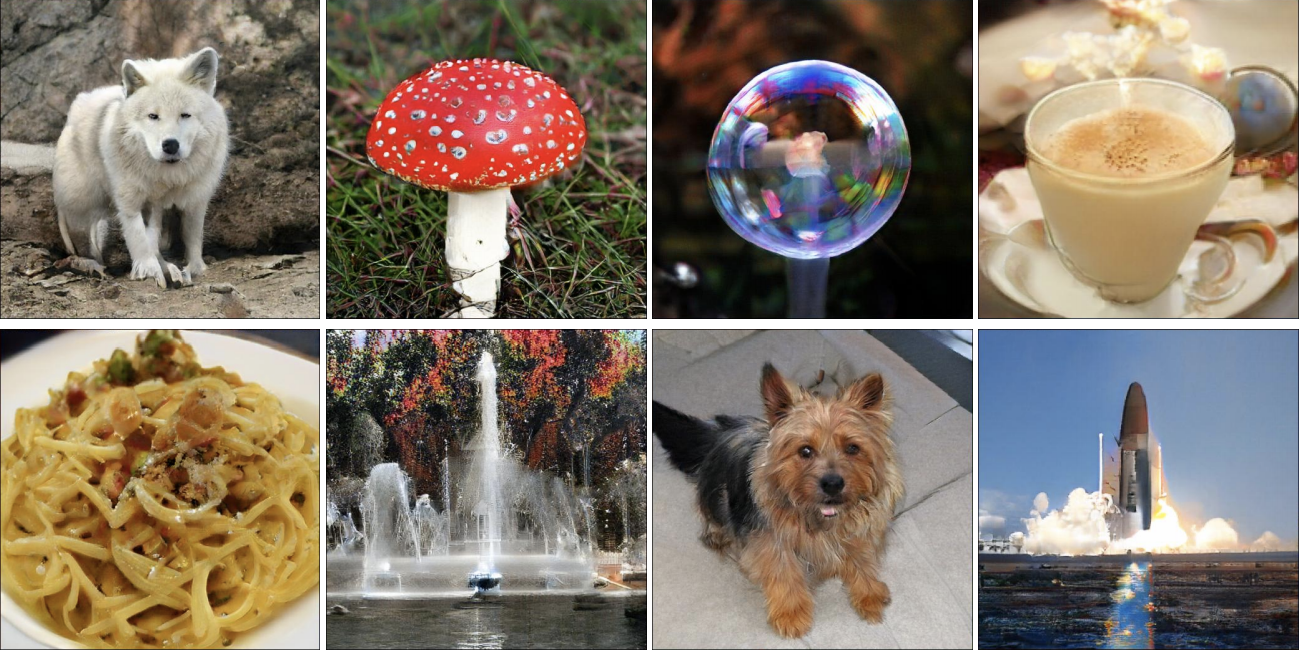
Preprints of the BigGAN paper are here and here. It’s been causing a buzz in the machine learning community. For generated images, their 512x512 pixel resolution is high, and they scored impressively well on a standard benchmark known as Inception. They were able to scale up to huge processing power (512 TPUv3′s), and they’ve also introduced some strategies that help them achieve both photorealism and variety. (They also told us what *didn’t* work, which was nice of them.) Some of the images are so good that the researchers had to check the original ImageNet dataset to make sure it hadn’t simply copied one of its training images - it hadn’t.
Now, the images above were selected for the paper because they’re especially impressive. BigGAN does well on common objects like dogs and simple landscapes where the pose is pretty consistent, and less well on rarer, more-varied things like crowds. But the researchers also posted a huge set of example BigGAN images and some of the less photorealistic ones are the most interesting.
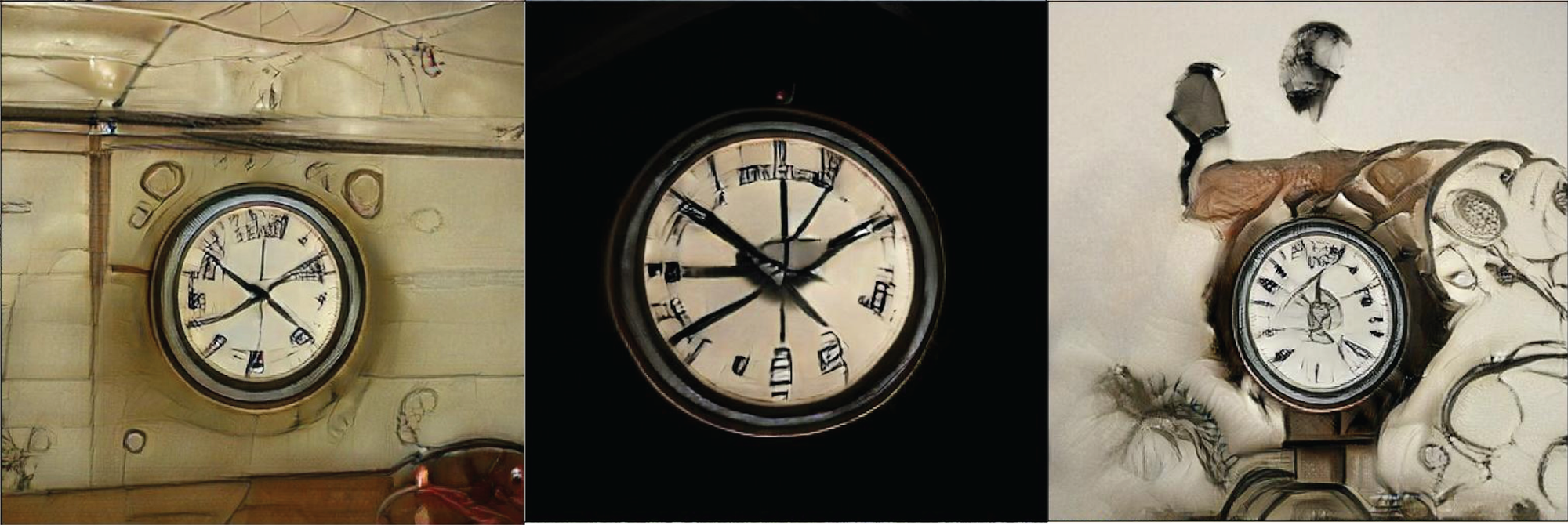
I’m pretty sure this is how clocks look in my dreams. BigGAN’s writing generally looks like this, maybe an attempt to reconcile the variety of alphabets and characters in its dataset. And Generative Adversarial Networks (and BigGAN is no exception) have trouble counting things. So clocks end up with too many hands, spiders and frogs end up with too many eyes and legs, and the occasional train has two ends.
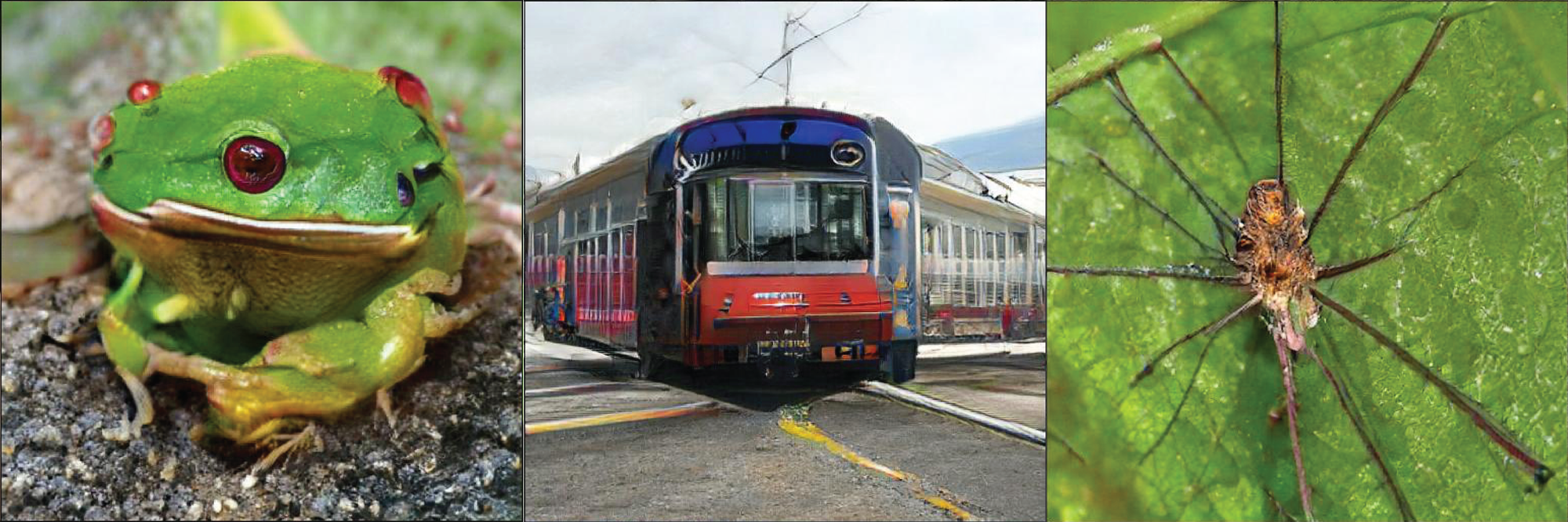
And its humans… the problem is that we’re really attuned to look for things that are slightly “off” in the faces and bodies of other humans. Even though BigGAN did a comparatively “good job” with these, we are so deep in the uncanny valley that the effect is utterly distressing.
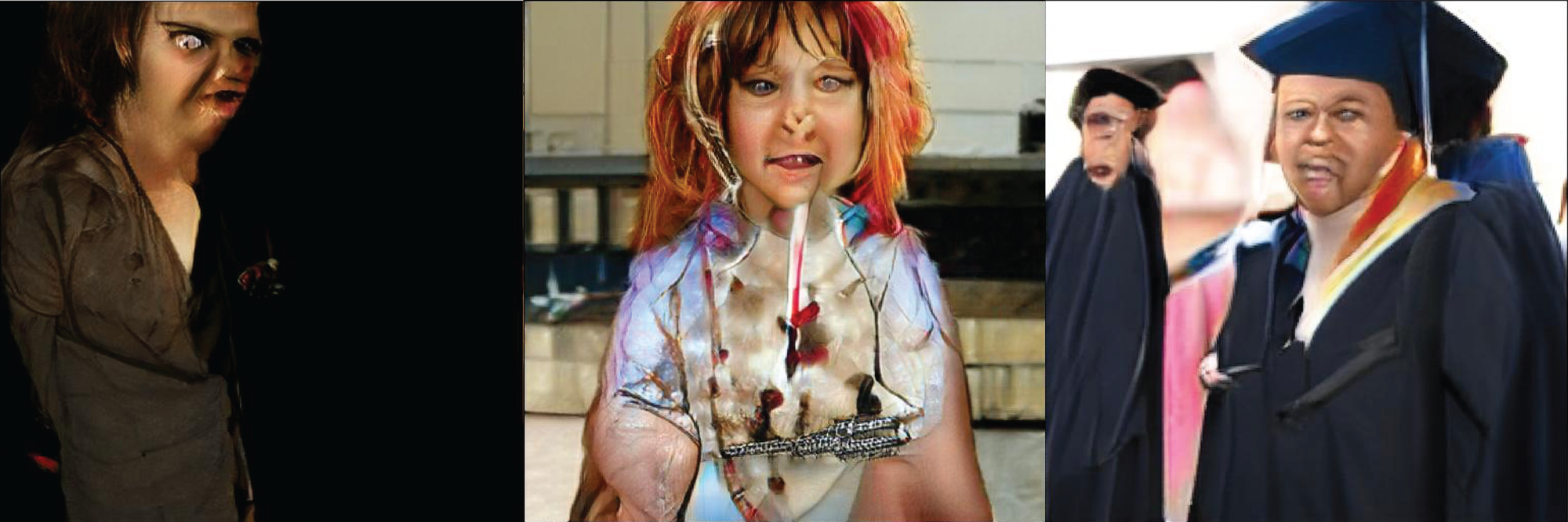
So let’s quickly scroll past BigGAN’s humans and look at some of its other generated images, many of which I find strangely, gloriously beautiful.
Its landscapes and cityscapes, for example, often follow rules of composition and lighting that it learned from the dataset, and the result is both familiar and deeply weird.
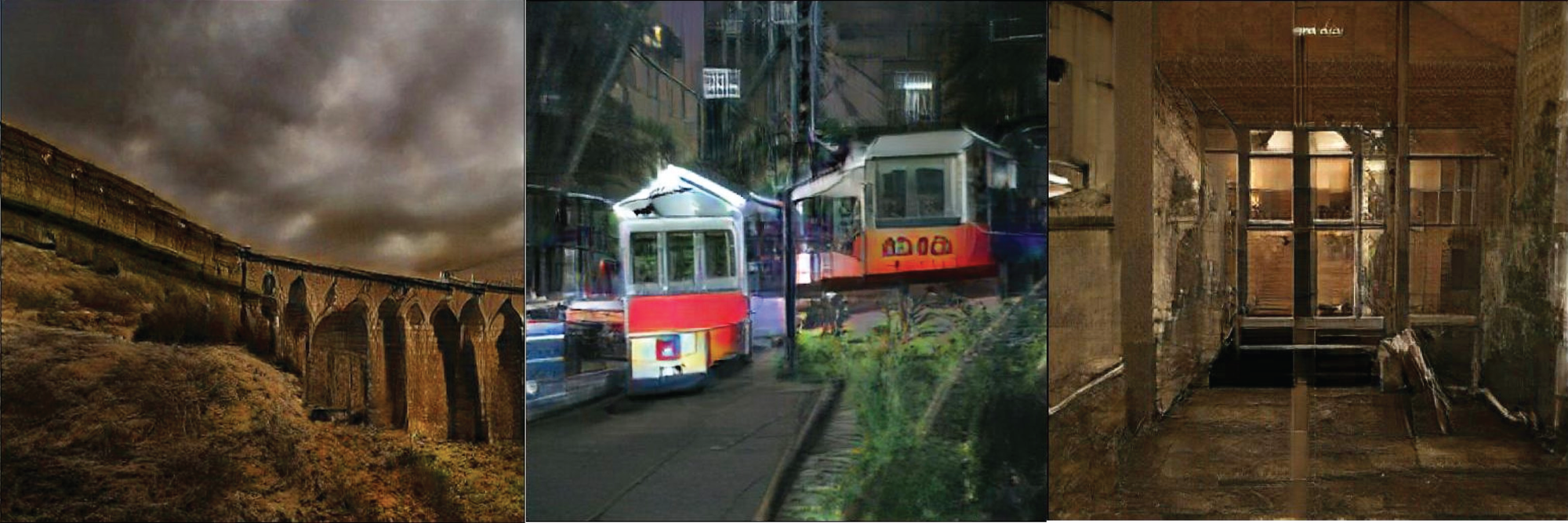
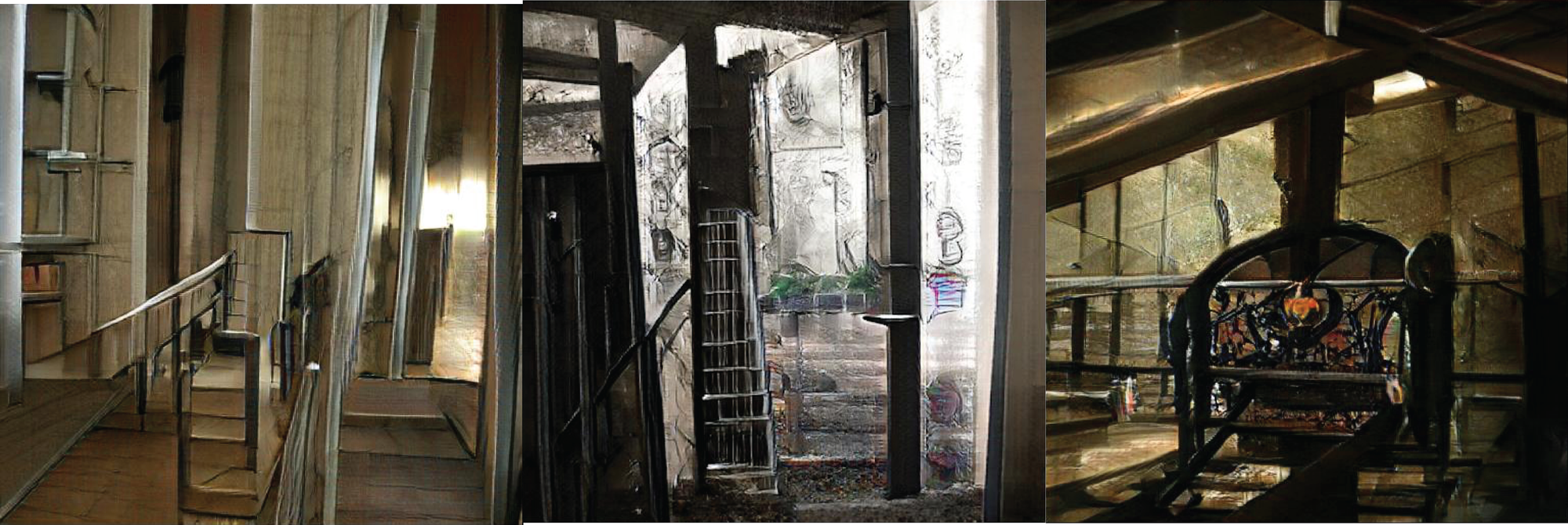
Its attempts to reproduce human devices (washing machines? furnaces?) often result in an aesthetic I find very compelling. I would totally watch a movie that looked like this.
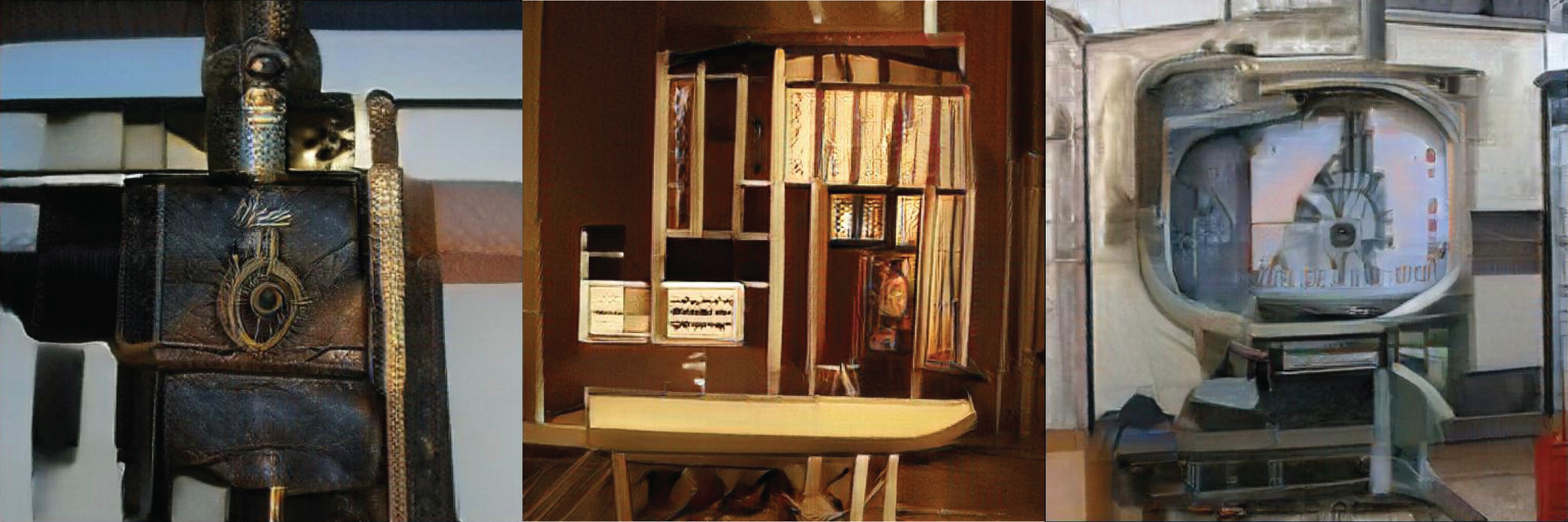
It even manages to imitate macro-like soft focus. I don’t know what these tiny objects are, and they’re possibly haunted, but I want them.
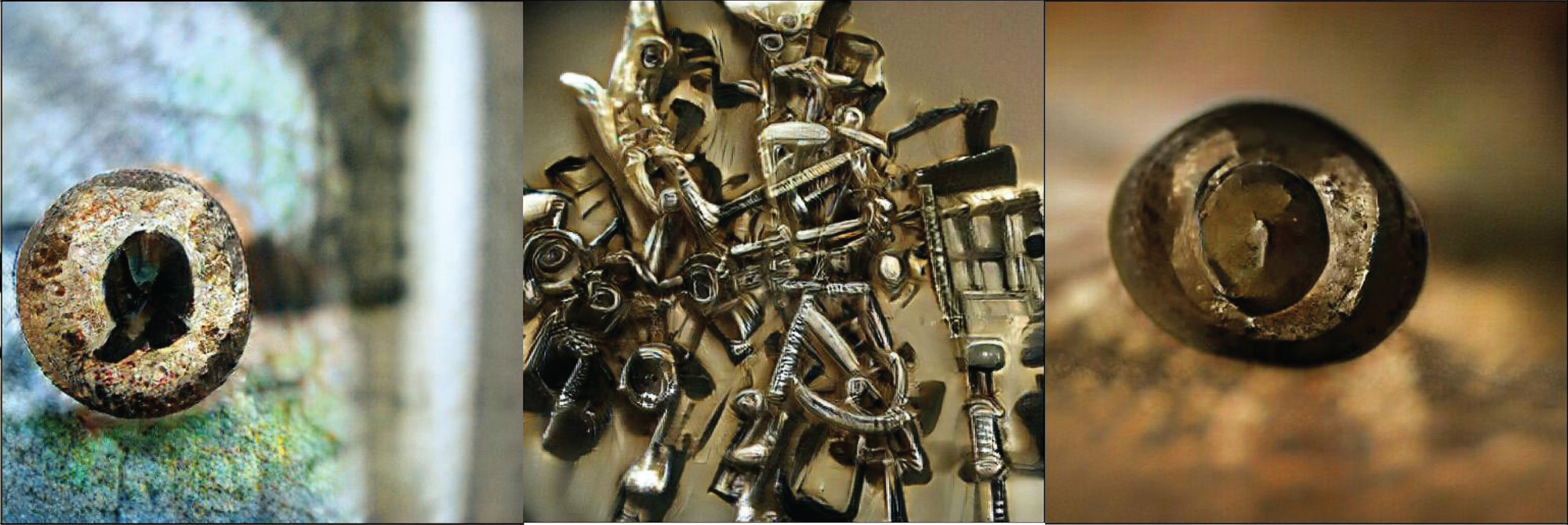
Even the most ordinary of objects become interesting and otherworldly. These are a shopping cart, a spiderweb, and socks.
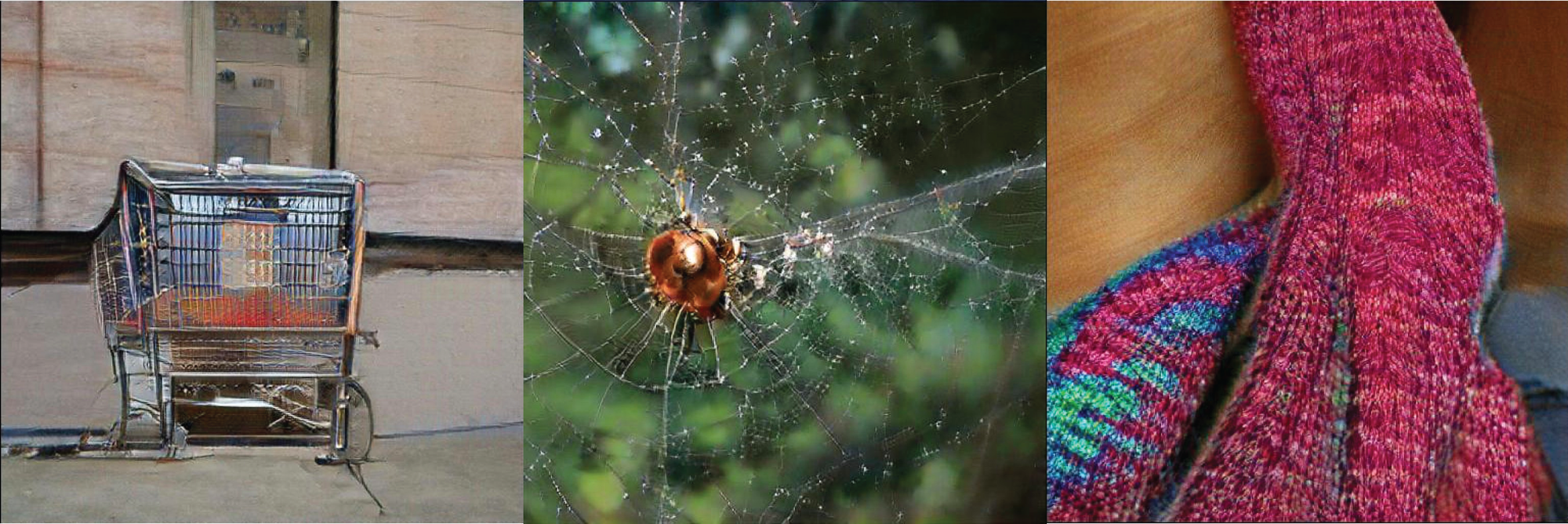
Some of these pictures are definitely beautiful, or haunting, or weirdly appealing. Is this art? BigGAN isn’t creating these with any sort of intent - it’s just imitating the data it sees. And although some artists curate their own datasets so that they can produce GANs with carefully designed artistic results, BigGAN’s training dataset was simply ImageNet, a huge all-purpose utilitarian dataset used to train all kinds of image-handling algorithms.
But the human endeavor of going through BigGAN’s output and looking for compelling images, or collecting them to tell a story or send a message - like I’ve done here - that’s definitely an artistic act. You could illustrate a story this way, or make a hauntingly beautiful movie set. It all depends on the dataset you collect, and the outputs you choose. And that, I think, is where algorithms like BigGAN are going to change human art - not by replacing human artists, but by becoming a powerful new collaborative tool.