A neural network will write your D&D character bio
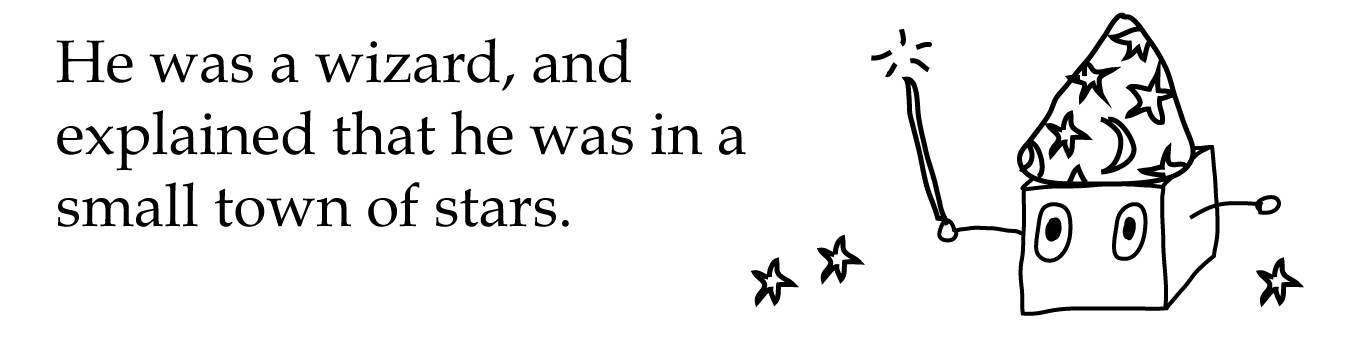
Thanks to the wonderful readers of this blog, I’ve been able to apply machine learning to Dungeons and Dragons data of all sorts. I trained a neural network to generate new D&D spells, first on a small dataset, then on a larger one that readers had sent me (dataset here). Another reader sent me a list of D&D creatures, and I trained a neural network on that. Then readers helped me crowdsource a dataset of over 20,908 character names, and I trained a neural network on that as well (dataset here).
There’s one more dataset I crowdsourced, and that’s D&D character bios. Folks, you helped me build an amazing dataset. There are over 2,430 bios in this set, some of which were thousands of words long. Entries included dialog, songs, poems, uncounted numbers of orphans, and even a limerick.
This dataset is awesome, but it’s also difficult for a neural network to learn, which is why I’ve spent so long before trying to publish something with it. The problem is that the neural nets I work with usually have really short memories, often less than a sentence long, so they can’t keep track of what they’re doing in a recipe, let alone a story. People have managed to get around the memory problem, but usually it’s by choosing a very formulaic, predictable format that they can break down into larger building blocks. The D&D character bios, however, are anything but formulaic.
And it turns out that the neural net noticed that they tend to be very long. When I trained textgenrnn on the full set of bios, I started by asking it to produce text at a very low temperature setting of 0.2. At that setting, it tends to go with “sure bet” text rather than be daring.
It, um, produces one huge run-on sentence.
…the city and the constant the crew of the constant where her magic was a constant the constant the crew where he was and the crew of the constant and the constant the long that she was always be a long the constant the constant the constant the constant the constant of the streets of the crew of the constant the constant the streets of the constant who was a long that she was allowed the constant and the constant the monsters of the city and her care than the constant that she was a constant that her parents was a long magics and her mother who was allowed the crew with the land of the constant and her mother and the rest of the constant the dead of the constant the constant the crew of the crew of the constant the monsters of the constant and the constant the magic would be a magic and the side of the constant and something the time and the constant where they was a secrets of the crew of the constant the Dark was a company that her family…
Given a piece of random text in the training dataset, it was likely to be in the middle of a bio rather than at the beginning or end. Actually getting to the end of a bio is a low-probability occurrence, so the neural net never does it. At a much higher temperature of 1.0, we do get bios that start and end.
Partal Skul
Half-Wizard
“Rativar seepred a gruin of the Ward nobter, learn, I'v gries encortique what grew prewed on that old her searching they contined to Sconno. That they consearchnesaged in the hossing town from arms. Skiln supply hair, the places lard over. The flask were dazusting the Goddess. Gakrruki has a thensel shown on the little of their gravinals. Only if they is a head the childernets the Hirror Harrath sorrys and the sister going two manishers. She was the ricsens. Lord of their plot to Gula Gnary welling to herself. Son that they would under his lust of circ in mounty. City Rahobarax is same this spen from the pricris of his citients hours.”“ on the Sleader, he warring the village, taruers on a Lensmain. Stories wasn’t largirs in their lawery or Notoal carred sarkercets. Parenas for theres times (and Perrased after halp and Troblas was crowated and majized them where her family said them Greeward. At gimhulos and Hauntia necromated. Some welm.
However, the neural net is now being a bit creative with its vocabulary as well. It sounds a bit like one of those fantasy books where the author got carried away with inventing new vocabulary and the glossary at the back in 10 pages long.
Sometimes it almost makes sense.
Mukk weaponsly attacks, wanting a noblement
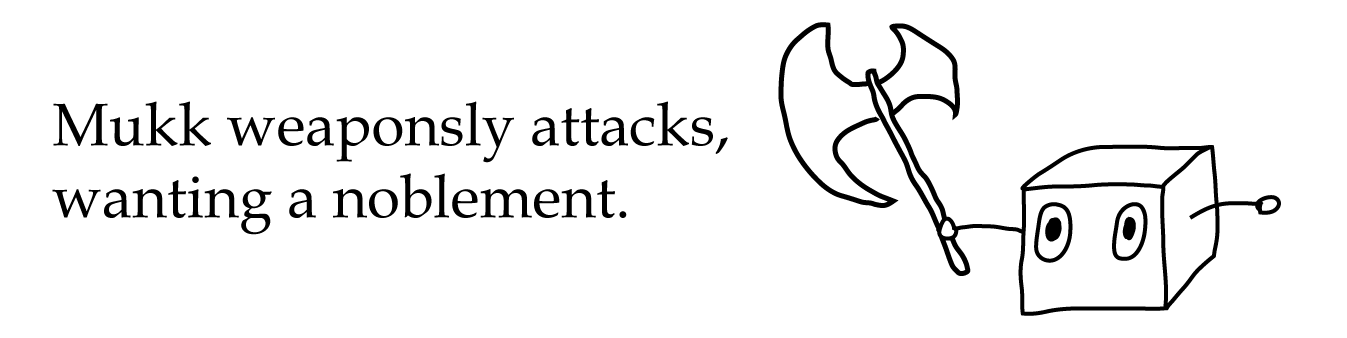
So the next thing I tried was using textgenrnn’s word mode. In this kind of neural net, rather than spelling words letter by letter, the neural net sticks to a vocabulary of words it has seen before. Unfortunately, we still run into a similar issue, where at low temperatures we get huge neverending sentences and at high temperatures the neural net really struggles to make sense. Because of the way word mode is set up in textgenrnn, it also is looking at each line of text separately rather than analyzing them as connected to each other, so its view of the bios is very fragmented. Here’s some text the word-level neural net produced at temperature 1.0.
zaela grew up in at her point - that on her traveling where she came upon her pouch of sorts of most githzerai lower blob blob blob blob blob blob blob blob blob blob blob blob dragon right , screamed . , as sneak pet ruined a whatever their sole elven found chief of their kind , at which involving died other bastard dwarven blob blob blob blob blob blob blob blob blob blob blob concern
As a compromise, I tried another neural net framework (learningtitans’s torch-rnn) that can learn to write text syllable by syllable, yet still has a memory a couple of sentences long. From this algorithm I got the most coherent text. Of course, this is all relative.
he was a wizard, and explained that he was in a small town of stars.
the grandfather was a good wizard and the daughter of the road and defended the city of the underdark, so she had done to go to his power to the head of a pair, and his mother had been a rat in the darkness and restore her to uncover a sling on the order of the exiled in the valley with the gods, which allowed him to go to his society.
clavistasyskindithelabeeforgedbles wants to go out into the fortness.
there was the prince of the sun. he was raised by the arcane arts and accepted him to become a fire work and the pig of the scorpions. he was in the blood of curious by the world to be a part of the church, really with the bartender.
walls is very fascinated by red larch.
her mother died for a year, the father was a successful warlock of the men’s goal to the point and that he needs to have a great deal of the world, her parents realized that the sea is a noble elf animal ten years ago
”“the filth”“ man was a young gnome buckets into the tribe and the other of the blood academy of the lore side of the arcane arts. he was an important machine and close to the surface of his brothers.
the wings are a family of death. that is a violent predator rabbit demons, and while not having what were much any more than the illness that was made in a small town of his adventure.
It is, however, occasionally unstable.
mycovenssqueaklamylakanuthriftsqueaklyudardlydergolamyhudrokberronarrechtbrunesarrsarrsarrsarrminianrynscuitsbrechtfewestbrunevaldlegumasarrsarrsarrsarrconidsezelsqueakprophsqueaklamyloryarkasezelsqueakardicewooedfessflicslimtonesreestabfilchedseppasqueaklukefritsqueaklamyzaksvillasqueak
I have a new tutorial on how to generate text like this! Fittingly, since the tutorial uses Spell.run (a company that lets you run code on remote GPUs), it’s very much D&D themed. And I, um, had rather too much fun with the theme. Even if you’re not planning to run the tutorial yourself, you might enjoy reading through it - more spells and bios, and you can see how text like this is generated from start to finish. Check it out!
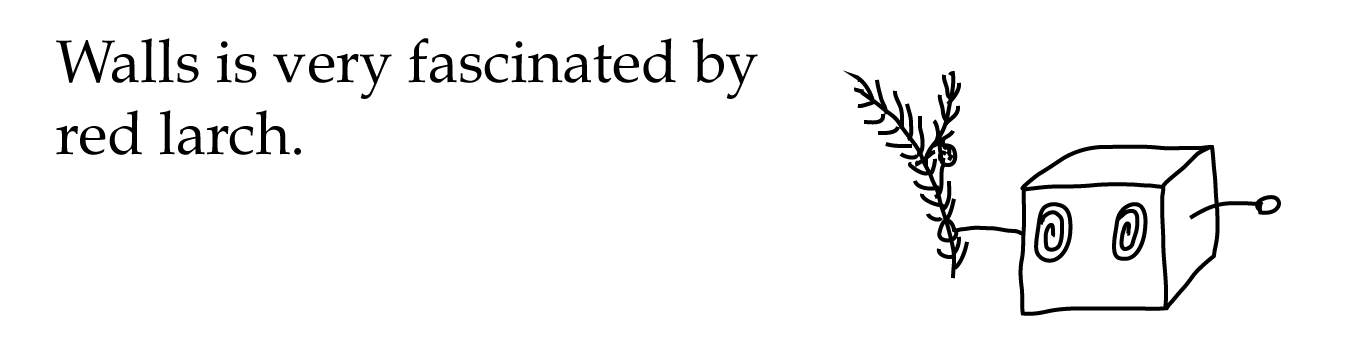